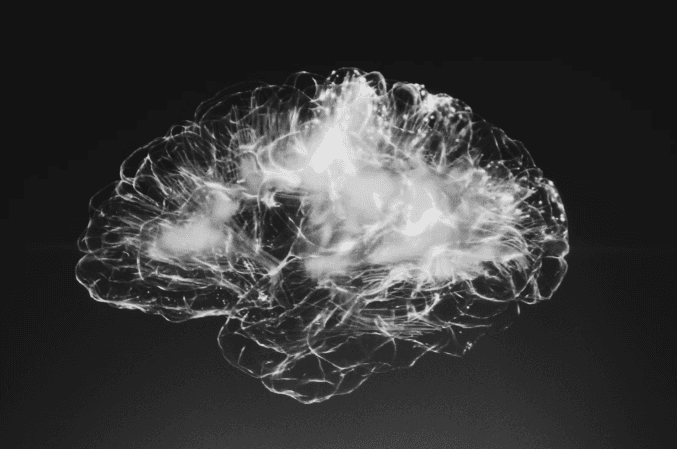
Bridging the Gap: Ai and Neurology in the Era of Precision Medicine
BA
Billal Alamarie
4 min readThe rise of artificial intelligence (AI) in various fields has led to remarkable advancements and transformations. One of the most significant areas is in the field of neurology, where AI holds enormous potential to revolutionize care delivery and patient outcomes. The integration of AI into neurology promises a future where we can anticipate disease progression, tailor interventions, and even uncover patterns invisible to the human eye. As we navigate this era where milliseconds...
Create an Account to Continue
You've reached your daily limit of free articles. Create an account or subscribe to continue reading.
Read-Only
$3.99/month
- ✓ Unlimited article access
- ✓ Profile setup & commenting
- ✓ Newsletter
Essential
$6.99/month
- ✓ All Read-Only features
- ✓ Connect with subscribers
- ✓ Private messaging
- ✓ Access to CityGov AI
- ✓ 5 submissions, 2 publications
Premium
$9.99/month
- ✓ All Essential features
- 3 publications
- ✓ Library function access
- ✓ Spotlight feature
- ✓ Expert verification
- ✓ Early access to new features